Meta has created a new generative AI for speech called Voicebox that can take an audio sample of just two-seconds and generate an authentic-sounding voice to deliver contextually accurate speech – outperforming Microsoft’s new text-to-speech (TTS) AI, VALL-E, by a significant margin.
Citing the risk of misuse, Meta is not publicly releasing the model or code.
That's despite CEO Mark Zuckerberg’s commitment to open-source AI, in the wake of its well-received release of the LLaMa group of large language models in February 2023, under a non-commercial research licence.
(The potential for fraud in synthetic “vishing” attacks that use the convincing sample of a CEO’s voice for example to persuade an unwitting employee to take actions in the interest of cybercriminals is colossal.)
The company also said that a model it had created alongside Voicebox can accurately distinguish between real and synthetic speech generated using the AI: “We also plan to investigate proactive methods for training the generative model such that the synthetic speech can be more easily detected, such as embedding artificial fingerprints that can be trivially detected without hurting the speech quality” its researchers said.
Join peers following The Stack on LinkedIn
They cited a 2021 paper by Ning Yu and peers in which the researchers embedded artificial "fingerprints" into image recognition training data using steganography. This resulted in the "surprising discovery" that the "same fingerprint information that was encoded in the training data can be decoded from all generated images" to achieve "deepfake attribution."
("Instead of encoding information into pixels of individual images, our solution encodes information into generator parameters [so] the generated images are entangled with that information" Yu wrote. "Compared to the pipeline of a generator followed by a watermarking module, our solution introduces zero generation overheads, and obstructs adversarial model surgery that targets to detach watermarking from image generation.")
Without going into detail, Meta's researchers suggested that in future similar techniques could be deployed to help identify convincing but synthetically generated speech from TTS engines like Voicebox.
Meta's new AI Voicebox: The use cases
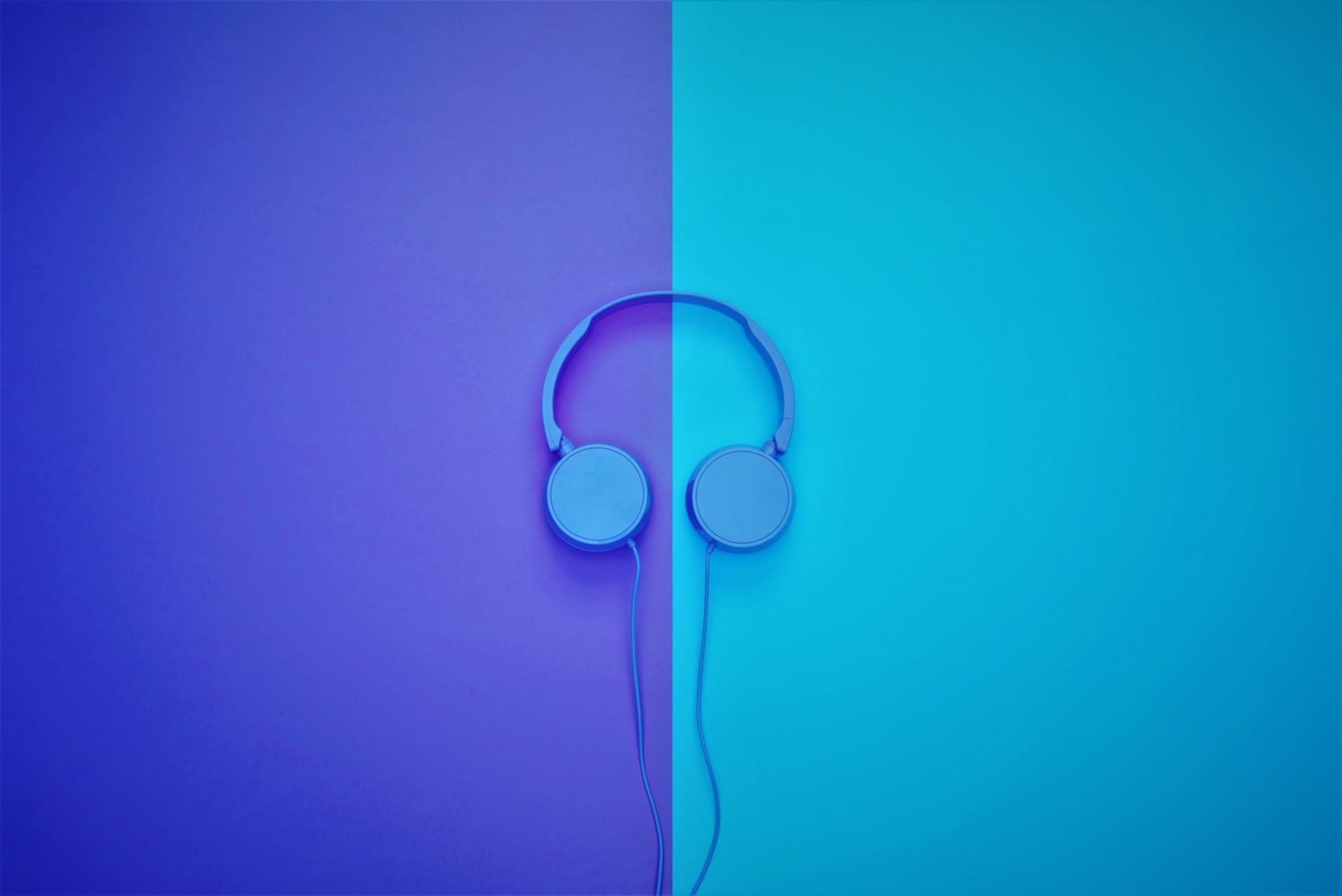
Voicebox was trained with more than 50,000 hours of recorded speech and transcripts from public domain audiobooks in English, French, German, Polish, Portuguese, and Spanish. It could be used to create customised voice chat assistants or game characters, Meta said, as well as to "resynthesize the portion of speech corrupted by short-duration noise."
Perhaps more usefully in future for enterprise users, its ability to rapidly generate speech that is representative of how people talk in the real world, it could be used to generate synthetic data that can help with the training of speech assistant models; existing experiments to this end suggest just degradation error rates of just 1% versus real speech, as opposed to 45% -70% degradation with synthetic speech generated by earlier TTS models.
"Zero-shot TTS could bring the voice back to people who suffer from diseases or underwent surgeries such as laryngectomy that causes inability to speak [and] be combined with visual speech recognition systems to avoid the need of typing. When paired with speech translation models, cross-lingual zero-shot TTS enables everyone to speak any language in their own voice," the 11 Meta researchers said in a pre-print paper.
More technically, in terms of modeling, Voicebox is a "non-autoregressive (NAR) continuous normalizing flow (CNF) model. Similar to diffusion models, CNFs model the transformation from a simple distribution to a complex data distribution (p(missing data | context)), parameterized by a neural network," they added. "We train[ed] Voicebox with flow-matching, a recently proposed method that enables efficient and scalable training of CNFs via a simple vector field regression loss. In contrast to auto-regressive models, Voicebox can consume context not only in the past but also in the future. Moreover, the number of flow steps can be controlled at inference time to flexibly trade off quality and runtime efficiency..."
The limited release on June 16 comes after (as The Stack first reported) Meta revealed plans to spend an eye-watering $33 billion this year to support “ongoing build-out of AI capacity” as CFO Susan Li put it in April.