Every data scientist would agree that there is no such thing as too much data and most would warmly welcome more when presented with the opportunity, writes Adam Lieberman, Head of AI and ML, Finastra.
More data can potentially help create better models, but most projects face the problem of data scarcity and this issue will most likely remain prominent for some time. This is frequently an issue for financial services use cases, where datasets are limited to begin with, thus restricting their modeling potential.
One way to deal with data scarcity is to create your own. Sometimes, we simply cannot overcome the problem of needing more data. It could be that data collection is too expensive or the data is not possible to collect in a reasonable time frame. This is where synthetic data can provide real value.
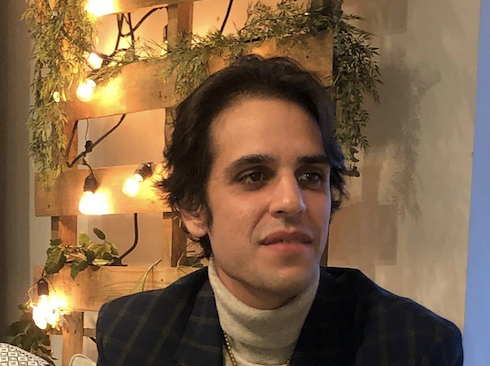
What is Synthetic Data
So what is synthetic data and how can it help?
Synthetic data is information that is generated artificially rather than produced by events in the real world.
This data, created by statistical techniques, allows us to generate new data points that are statistically sound relative to our real data. Here, we can arrive at high quality pseudo-production or operational data that we can leverage to train our statistical models.
See also: The NHS data strategy: 5 things to know, from data sharing, to OSS, and synthetic data.
One example of this could be a model that is centered on predicting the probability of small and medium-sized businesses (SMBs) in the retail sector defaulting on their loans.
For this example, the conditions of the components such as location, turnover and number of employees, are universally known, reducing the possibility for anomalous components.
SMBs, by rule of thumb, can’t employ more than 250 employees and are also unlikely to be citing a turnover in the billions, meaning the synthetic model wouldn’t create such data points. The synthetic model is then able to learn the statistical nature of the listed components and create new data that blends into the real datasets. The model can then be expanded and used to train an advanced loan default prediction model.
For data scientists, the main criteria for data is to create balanced, unbiased, accurate and high-quality models. If the data is doing this, then for the developers, an expansion of our dataset to include synthetic data can potentially help us achieve our model’s performance goals.
Digital Growth of Financial Services
Financial services is becoming increasingly digital. Consumers are steadily building up their digital footprint through engagement and interactions with products and services online.
This creates large datasets that hold the essence of a consumer’s identities and behaviors. Much of the data is sensitive and there are many legal barriers to sharing datasets. It is therefore crucial that this data is protected.
Yet this data also holds the power to support innovation throughout the industry, helping to advance data and analytical modeling to improve decision-making, user personalization and automation. This then has a more significant impact, where financial services institutions can use such data to help identify how best to target the delivery of social benefits such as financial inclusion and financial literacy, as well as how to recognize patterns in fraudulent behavior to assist in preventing financial crime.
See: The UK's FCA wants to learn from synthetic data experiences…
Leveraging synthetic data is one way we can protect consumers’ data whilst striving for innovation in the industry. Synthetic datapoints feel real but do not relate to real accounts and individuals.
However, synthetic data is still in its infancy of development, with promising growth and use cases. Ground-breaking techniques for synthetic data generation are in the works, with ongoing research into the level of privacy risk that correlates to the datasets, looking at the possibility of anonymizing a real consumer that is mixed within the synthetic population in specific situations. Advances in technology and computational power have also led to improving synthetic data accuracy and stronger guarantees around privacy protection.
Among developers, synthetic data is considered one of the most promising tools for sharing data where privacy is a priority, making it a viable tool for training AI models in environments with strict data privacy rules.
The Future of Synthetic Data
The use of AI and ML has the potential to disrupt financial services. Research generated by Nvidia, outlined that 81% of C-suite respondents in financial services saw AI as important to their company’s future success, and more than half thought it gave them a competitive advantage. And synthetic data is an important tool to truly generate advances with AI and ML for progressive disruption.
The potential for synthetic data to alleviate the data-access challenge for innovation is vast.
Access to readily available synthetic data from third parties such as RegTechs and B2B Fintechs could allow the construction of potentially better models. It can also aid in revealing trends, patterns and insights that are tricky to spot in limited real-world datasets. Largely, synthetic data provides a crucial step for developers in their battle to innovate and develop varying models. Through collaboration and sharing, synthetic data is a powerful tool with an extensive research community behind it, with the goal of helping developers access more data quickly and easily to innovate industry-wide and develop higher-quality models.